How was this content?
Spotlight: Datagen Creates High-Fidelity Synthetic Data to Address Human-Centric Problems
When Gil Elbaz and Ofir Zuk founded Datagen in 2018, it was with the purpose of re-inventing the broken process of how clients obtain data for computer vision network training. More specifically, they wanted to bring data simulation to every computer vision team in a continuous and scalable way.
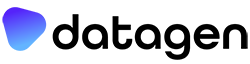
Because AI model performance relies on both the quality of the model and the quality of the data used to train it, it’s essential to have a large quantity of good data—and it’s often challenging to collect as much as needed. Real-world data also tends to be problematic when it comes to speed of acquisition, precision, expense, and bias. “Someone will collect [real-world] data of different identities—for example, for faces—and they will not collect enough of certain ethnicities, or ages, or gender,” explains Shay Navon, Datagen’s Senior Product Marketing Manager. “And then you get this bias.”
In order to help computer vision teams fight bias, Datagen offers a unique way to generate data using computer algorithms. Its synthetic data is akin to real-world data both statistically and mathematically, but can be generated quickly, with less expense, and is exempt from human error. Instead of tasking a human with the chore of gathering and annotating data manually – a labor intensive task—which requires them to take a photo of a face and then label its features by hand—the synthetic data is generated on a massive scale, with built-in ground truth annotations , such as eye direction, that would be impossible for a human to determine. The result is more accurate and detailed data annotation without the challenge of manual tagging.
“We are simulating the world to bring AI to production faster,” says Karine Regev, Datagen’s VP of Marketing. “Bringing AI to production is by itself an unsolved challenge for most companies out there, so we are making it more professional, more accurate, solving problems like privacy, solving problems like bias in the data which are the largest bottlenecks in modern AI.”
Datagen offers clients a self-service platform that uses 3D simulations to train their algorithms. “In order to train a model, you need millions of different images,” says Regev. “And this is exactly where we fit in. [Datagen customers] have the ability to control the scenes, the ability to control the background, the different modalities, the different labels that you need, the lighting, the gender, ethnicity, everything.”
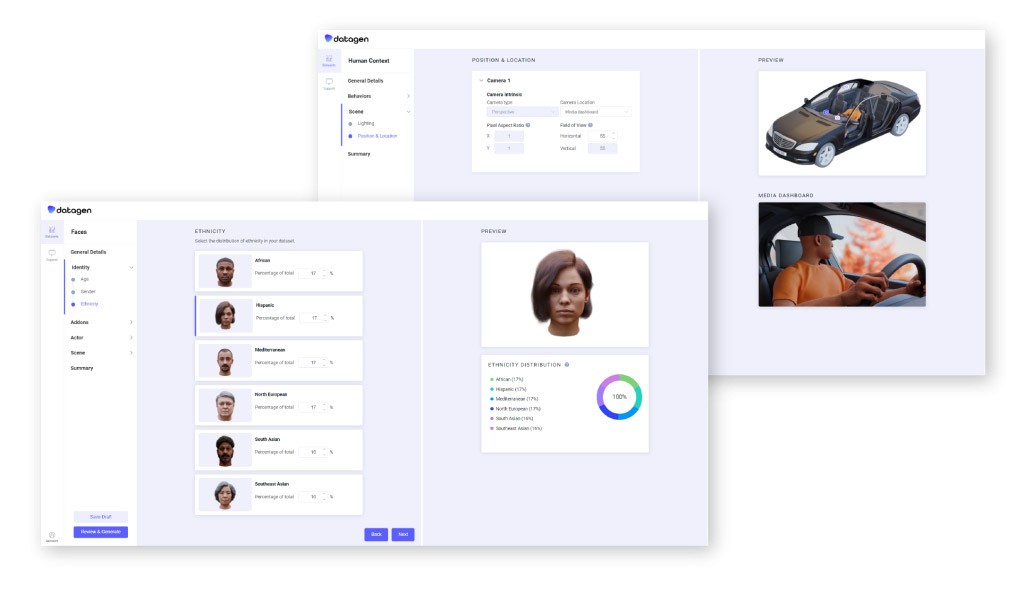
In addition to generating diverse data that looks real, scales, and is pixel-perfect, Datagen offers its customers complete confidentiality. “It’s fully privacy compliant, as the data contains zero PII (Personally Identifiable Information),” says Shay Navon about the synthetic data. “Nobody can say, ‘This is someone that we are using that is a problem privacy-wise.’ Our human-centric expertise and data focuses on several domains, from facial landmarks detection, gaze estimation, and expression analysis to full human body poses, body parts like eyes, hands, etc.”
In the very near future, it’s predicted that it will be more common to train models with synthetic data than to collect it from real-world sources. In accordance, Datagen has been growing rapidly, expanding from around 40 employees to nearly 100 over the last nine months. “We are working with some of the largest tech companies in the world in different verticals,” says Regev. “Solving different use cases from AR/VR/Metaverse to driver monitoring for in-cabin automotive, to home security and smart offices.”
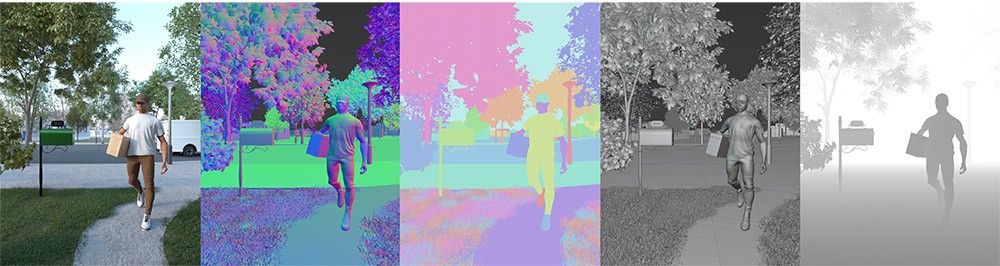
In order to meet this new demand, Datagen decided to switch to cloud architecture. Their priority was to scale using the latest GPU models. After an in-depth analysis of cloud providers, they turned to AWS, determined to develop their system on top of Kubernetes. Datagen designed a custom scheduling software system called Agni that integrates with Elastic Kubernetes Service (Amazon EKS) and uses Kubernetes auto scaling and AWS Auto Scaling Groups.
Agni—and the entire Datagen data-generation platform—now relies on CPU and GPU spot instances, which has helped them to reduce costs and build a more efficient system. It also enables them to maintain a relatively small system that can dynamically grow to hundreds of thousands of concurrent jobs and shrink on demand, resulting in a self-service platform hosted by AWS.
Looking forward, the Datagen team predicts that the need for synthetic data will continue to grow. “We’re seeing a lot of demand, both in the traction and prospects, the need for thought leadership, the need for technology, and a solution like ours that can actually lead the conversation when it comes to synthetic data,” says Regev.
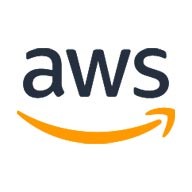
AWS Editorial Team
The AWS Startups Content Marketing Team collaborates with startups of all sizes and across all sectors to deliver exceptional content that educates, entertains, and inspires.
How was this content?